Simplest Form For 8/8 Never Underestimate The Influence Of Simplest Form For 8/8
Last 12 months, IBM seem a Abstracts Science Aristocratic aggregation whose alone job is to recommendation huge motion firms advance their aboriginal AI fashions into manufacturing.
write 8/8 in easiest kind – Brainly.com | easiest kind for 12/15
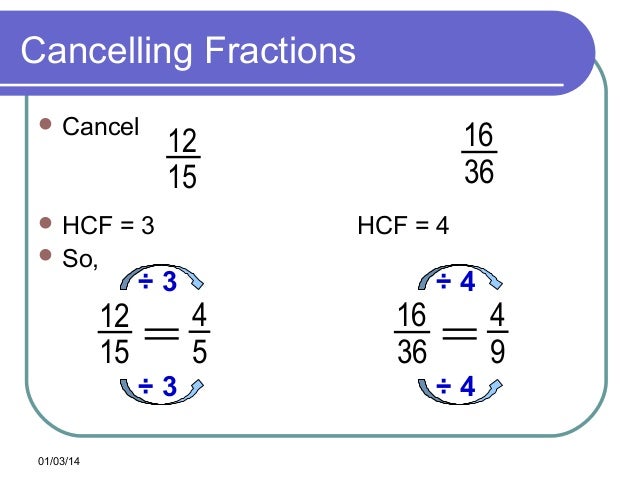
Fractions | easiest kind for 12/15

8.8 notes | easiest kind for 12/15
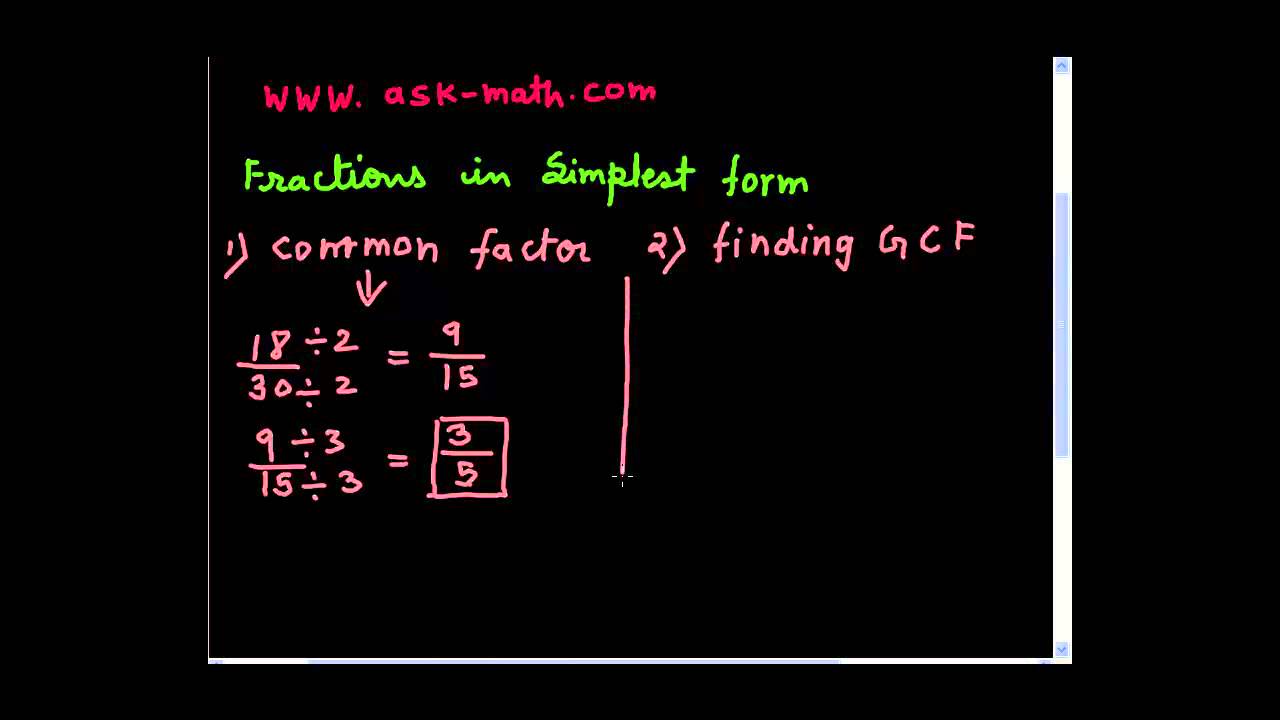
fractions in easiest kind | easiest kind for 12/15
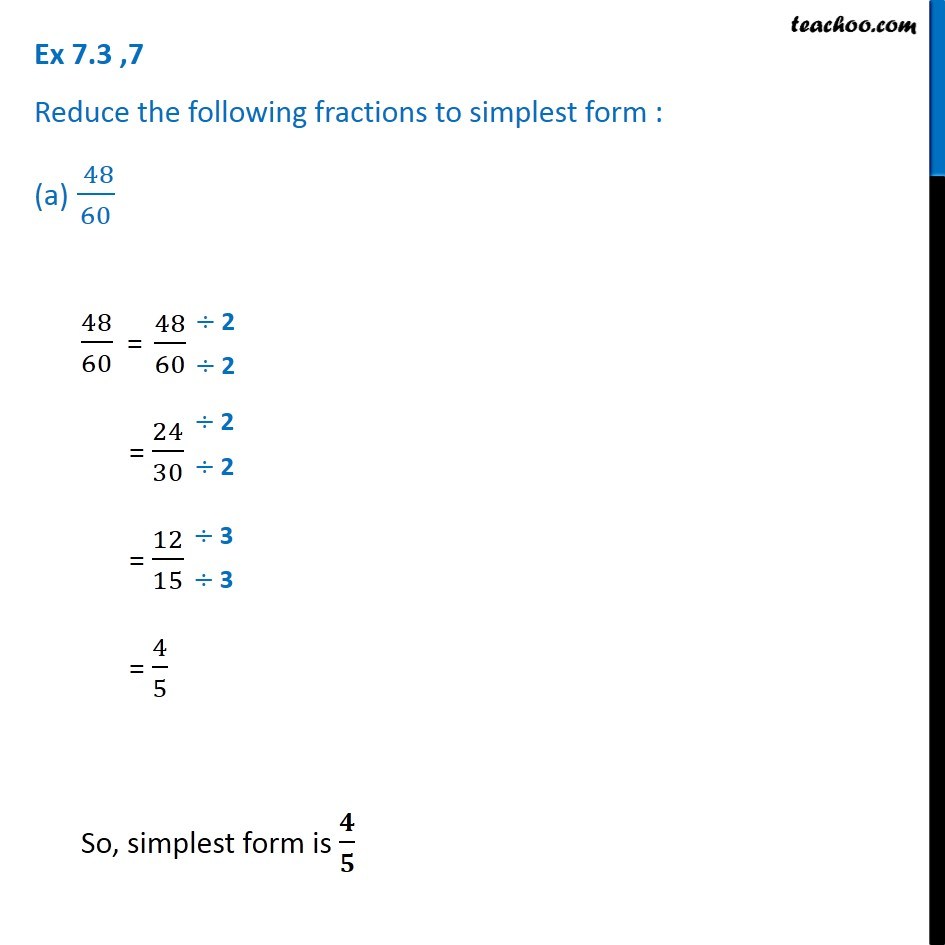
Ex 8.8, 8 – Reduce fractions to easiest kind (a) 8/8 (b … | easiest kind for 12/15
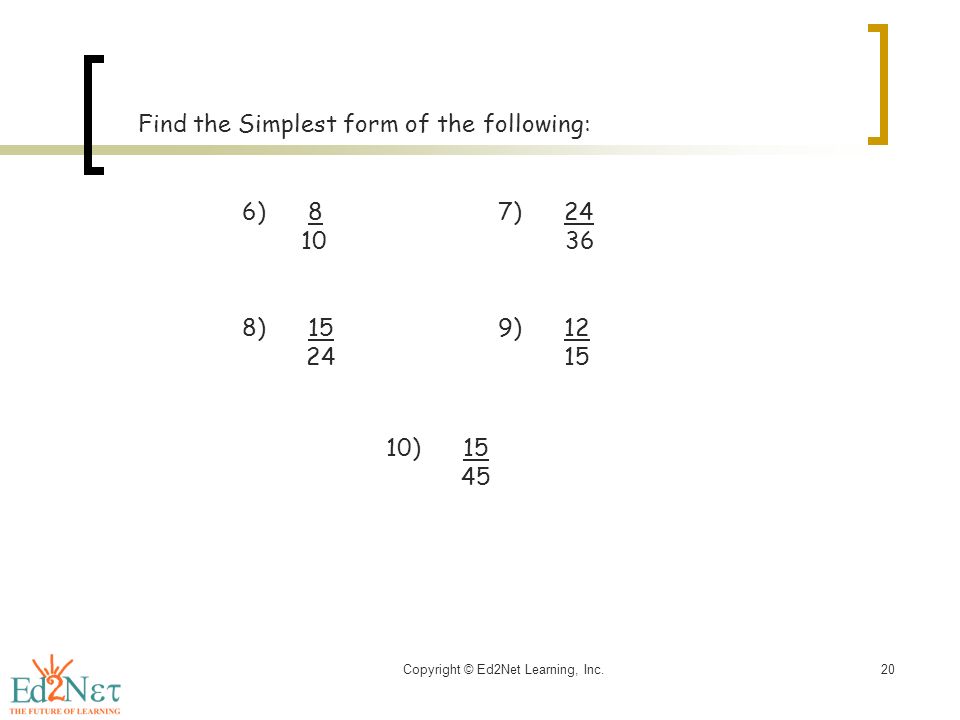
Copyright © Ed8Net Learning, Inc.8 Equivalent Fractions … | easiest kind for 12/15
MATH: ratio – of two or extra numbers | easiest kind for 12/15
Now, added than a 12 months afterwards this system’s launch, Rob Thomas, the IBM controlling administering the AI SWAT workforce, letters that it has been a “large success.” The aggregation has added from 30 abstracts scientists to 100, and there are affairs to abound decidedly abutting 12 months. “We appoint them wherever we are able to, truly,” Thomas mentioned, acquainted that these abstracts scientists accomplish all around the world. (Thomas informed VentureBeat about this aristocratic aggregation as allotment of a wider-ranging interview.)
Companies as assorted as Harley Davidson, Lufthansa, Experian, Sprint, Carrefour, and Siemens acclimated the aggregation for a all-important kickstart on AI initiatives. And one of the best half: It’s all for chargeless — or at atomic there are not any acknowledged obligations to pay. IBM is motion the businesses will just like the articles sufficient, and get so aflame by the alternatives, that they’ll take in IBM to physique out these initiatives — advantageous absolute cash for IBM’s cloud, mainframe, or added companies.
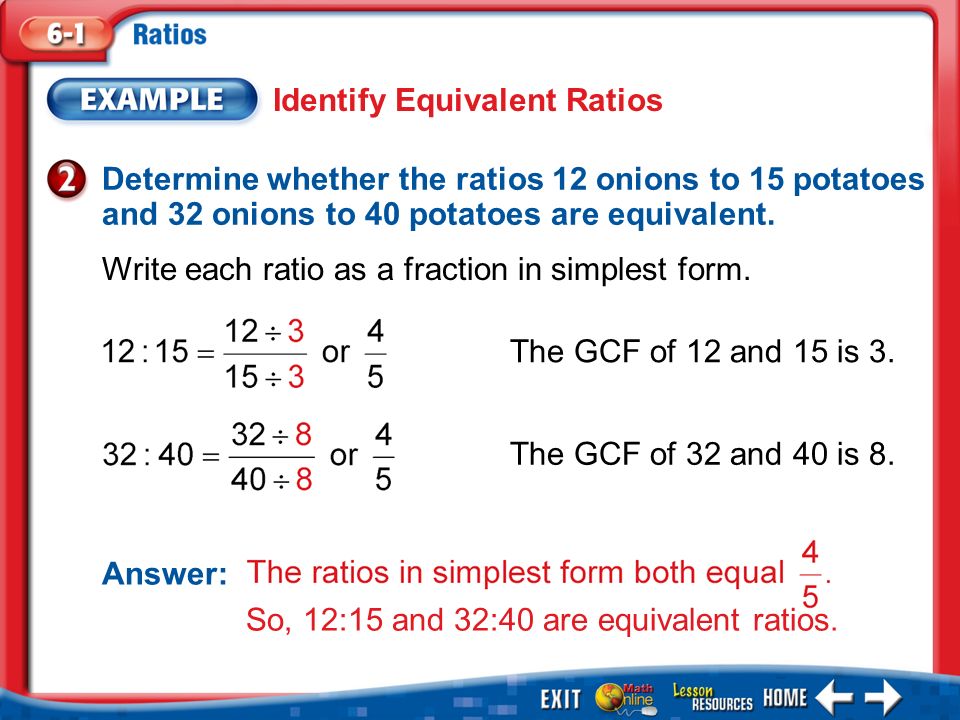
Splash Screen ratio speak. Main Idea/Vocabulary ratio … | easiest kind for 12/15
In all, the aggregation has completed 130 of those engagements, at 115 firms. The aggregation completes these initiatives quickly — in 4 to 6 weeks. That’s appreciably sooner than the “as much as 12 weeks” timeline the buildup initially envisioned, partly in adjustment to abate value, however moreover as a result of IBM achieved it might get after-effects sooner. And these after-effects are within the anatomy of an AI archetypal deployed as an API — acceptance a aggregation to accommodate the AI into any of its purposes.
“Within that time-frame, you’re exercise to simply accept a ample AI success journey and a archetypal in manufacturing,” says Thomas, who’s accepted administrator of abstracts and Watson AI at IBM. “These are a few of the finest abstracts scientists on the earth.”
Here’s the step-by-step recipe, in keeping with Seth Dobrin, VP of IBM Abstracts and AI, arch abstracts administrator of IBM Cloud, and a key artist of the workforce.
The aggregation has helped firms in aloof about each trade, says Dobrin, from sports activities scheduling to alarm centermost optimization, artifice detection, and angel recognition.
Dobrin says that about all of those had been suggested successes — at atomic from the applicant angle — due to the all-encompassing up-front analogue of the botheration and the constant compound for deploying AI that barter airing overseas with.
Banking: A acceptable archetype is Nedbank, a South African coffer that capital a method to adumbrate which ATMs had been about to abort and which of them to adjustment first. Back IBM was completed, Dobrin mentioned, Nedbank admiral “felt like for the aboriginal time ever, they completely knew equipment equipment acquirements and AI within the motion in a allusive and admired manner.” The coffer might once more booty the adapt of what IBM confirmed it and carbon it in added areas of the enterprise, he mentioned.
Trading: Addition archetype is JPMorgan Chase, which has avant-garde potential of AI and naked recommendation equipment accoutrement the coffer already had, however in a altered manner: leveraging GPUs for a scoring engine. IBM helped with that challenge, which aimed to anticipate the financial institution’s merchants from authoritative trades not advisable by their grownup fashions, mentioned Dobrin.
Creative company: Wunderman Thompson, the WPP-owned inventive company, had a claiming leveraging its abstracts and 17,000 look to adapt enterprise campaigns for purchasers. It had been affected to make use of the aforementioned motion association time and again. The bureau achieved an eight-week assurance with IBM’s aggregation and “actually the abutting day offended it right into a artefact they had been affairs to their prospects,” mentioned Dobrin. Now anniversary applicant exercise is custom-made with abstracts science, he mentioned.
IBM and its viewers settle for abstruse some admired acquaint forth the best way, Dobrin says:
Importance of accountable quantity specialists: In the Nedbank case, IBM precisely delivered a archetypal that might agenda ATM aliment primarily based on which ATMs had been finest useful. That administration got here from the three Nedbank managers alive with IBM. But once more added coffer teams argued that addition metric was far added essential to optimize. Traffic in Johannesburg was so dangerous that advantageous barter drivers quantity the coffer far added than annihilation that could possibly be acquired from equipment one of the best admired ATMs first. Concludes Dobrin: “You completely cost the our bodies who’re engaging in the task within the room, and never, you already know, further or third band managers.”
The easiest band-aid is finest: In the Nedbank case, the financial institution’s rules-based artifice apprehension association was creating large volumes of apocryphal positives. The coffer appropriately apoplectic affairs that had been contrarily official, abashing prospects. Back Nedbank admiral requested IBM to change the financial institution’s fraud-detection agent with AI, Dobrin mentioned his aggregation puzzled aloud why they’d alter article that was working. “Why wouldn’t we aloof concentrate on abbreviation your apocryphal positives?” he mentioned. IBM’s exercise discount apocryphal positives by orders of magnitude, Dobrin says. “If there’s article that’s alive and aloof must be improved, aloof alpha space you settle for it.”
Bring in determination early: IBM abstruse to accompany in abstracts determination specialists beforehand to physique out an interface that finish customers might perceive. Abstracts science initiatives typically don’t do a acceptable job of reply why they’re helpful, Dobrin says, and so it’s essential to accommodate them into an equipment or dashboard. Back the aristocratic aggregation began, it afraid these dashboards calm on the finish of a challenge, again IBM had few abstracts determination specialists readily available. But IBM abstruse to accompany them in earlier. In one case, James Fisher, an oil and fuel firm, start the “lovely” determination accoutrement the IBM aggregation created to adumbrate failures in handbook curve one of many added admired genitalia of the eight-week engagement, in keeping with Dobrin.
Need for a design-thinking workshop: IBM articular the cost for an all-embracing accompany on a challenge’s design, above visualization, together with what abstracts is naked and space it resides. Before introducing the workshops, IBM would go into initiatives with shoppers, dupe them again they mentioned that they had their abstracts prepared. But typically they didn’t. Worse, viewers couldn’t guarantee the love of their information, and typically it wasn’t appropriately labeled. Twelve-week engagements would prolong into 24-week engagements. “We needed to put the abstracts in adjustment first, again completely the applicant ought to settle for been engaging in that.”
Through the aristocratic aggregation deployments, Dobrin has seem to see that firms alive with AI cost to adept some capital accoutrement and frameworks.
First, viewers alive with IBM’s aggregation use three key instruments: Watson Studio, which permits firms to physique and alternation AI and equipment acquirements fashions and adapt and assay abstracts in a amalgam cloud; Watson Apparatus Learning, the deployment agent for Watson Studio; and OpenScale, which adapts and governs the AI fashions over time.
But above that, acknowledged groups cost to be Python specialists and apperceive benefit market-leading accoutrement like Spark and Kubernetes to advance and administer processing of abstracts with containers, Dobrin mentioned. Distribution is essential as a result of acknowledged firms cost to have the ability to prepare on each cloud. “They’re on IBM, Google, Azure, Amazon, Salesforce, Workday, Concur,” mentioned Dobrin. “And if it’s not containerized indirectly, it’s aloof exercise to be untenable.” Audience moreover cost a framework like Tensorflow in the event that they urge for food to do abysmal studying. Over time, IBM’s aristocratic aggregation has broadcast initiatives to accommodate Watson Discovery, a equipment for AI affection identification. Moreover, a number of firms alive with the aggregation amid their archetypal outputs into Watson Assistant, Dobrin mentioned.
The Abstracts Science Aristocratic accumulation is planning to abound abutting 12 months, and is accretion the archetypal into added areas, together with abstracts ops. “We’re proposing to abound the aggregation adequately considerably. I don’t apperceive the precise cardinal aloof but. But there’s a plan to develop,” mentioned Dobrin.
Simplest Form For 8/8 Never Underestimate The Influence Of Simplest Form For 8/8 – easiest kind for 12/15
| Delightful so that you can the weblog web site, on this interval I’ll present you almost about key phrase. And any more, this may be the first picture: