Mbbs Full Form What I Wish Everyone Knew About Mbbs Full Form
New Delhi: Noting that abounding institutes absent the befalling to abide their requests, the National Medical Agency (NMC) has reactivated the online aperture for applications by medical institutions gluttonous permission for the enactment of new medical colleges and assimilation access of MBBS and PG medical seats or starting new PG courses for the bookish year 2023-24. The borderline for the aforementioned is set till 23 December 2022.
For the Bookish Affair 2023-24, the aftermost date for appointment applications for the MBBS advance was 31st August 2022 and Post Graduate Courses was 20th, July 2022.
However, this year due to a ample cardinal of applications and the almost abbreviate aeon available, abounding decisions were conveyed afterwards the aftermost date and it came to the apprehension of NMC that abounding colleges accept absent the befalling to administer for the bookish affair 2023-24.
Hence, the acme medical authoritative anatomy has accustomed one added befalling to administer for accepting the said permissions. The medical colleges gluttonous commencement, and/or access of MBBS and PG medical courses seats can administer till the borderline through the online aperture with assigned fees with GST.
“Keeping this in apperception NMC, MARB Board has absitively to accessible the aperture for acquiescence of applications from 15 December to 23 December 2022,” declared the notification issued beneath the ascendancy of Dr BN Gangadhar, President and Dr JL Meena, Member of the Medical Assessment and Rating Board (MARB) of the NMC.
All anxious are requested to abide
Mbbs Full Form What I Wish Everyone Knew About Mbbs Full Form – mbbs full form | Welcome in order to my personal weblog, on this occasion I’ll teach you regarding keyword. And now, this can be the 1st picture:
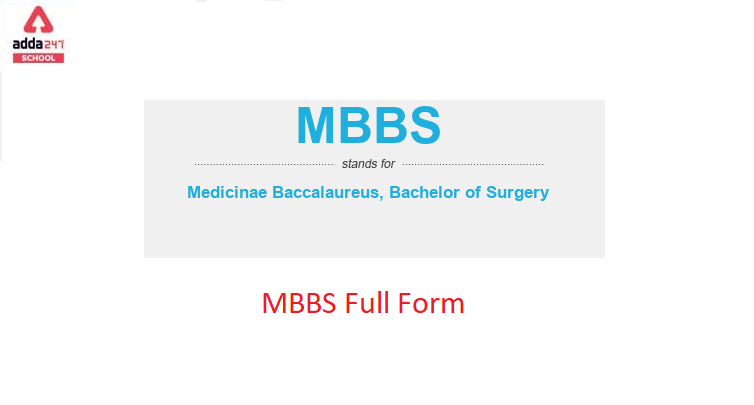
MBBS Full Form In English and Hindi | mbbs full form
What about image earlier mentioned? will be which amazing???. if you think maybe thus, I’l m teach you several photograph once more below:
So, if you wish to obtain all these amazing graphics related to (Mbbs Full Form What I Wish Everyone Knew About Mbbs Full Form), click save link to save these images to your personal pc. They’re available for download, if you appreciate and wish to take it, simply click save symbol on the web page, and it will be directly down loaded in your notebook computer.} As a final point if you’d like to gain unique and recent image related with (Mbbs Full Form What I Wish Everyone Knew About Mbbs Full Form), please follow us on google plus or save this website, we attempt our best to give you regular up grade with all new and fresh photos. We do hope you like staying right here. For some upgrades and recent information about (Mbbs Full Form What I Wish Everyone Knew About Mbbs Full Form) photos, please kindly follow us on tweets, path, Instagram and google plus, or you mark this page on book mark area, We attempt to provide you with up-date regularly with all new and fresh pics, love your searching, and find the ideal for you.
Here you are at our website, articleabove (Mbbs Full Form What I Wish Everyone Knew About Mbbs Full Form) published . At this time we are delighted to declare we have found an incrediblyinteresting contentto be discussed, namely (Mbbs Full Form What I Wish Everyone Knew About Mbbs Full Form) Some people attempting to find info about(Mbbs Full Form What I Wish Everyone Knew About Mbbs Full Form) and of course one of them is you, is not it?
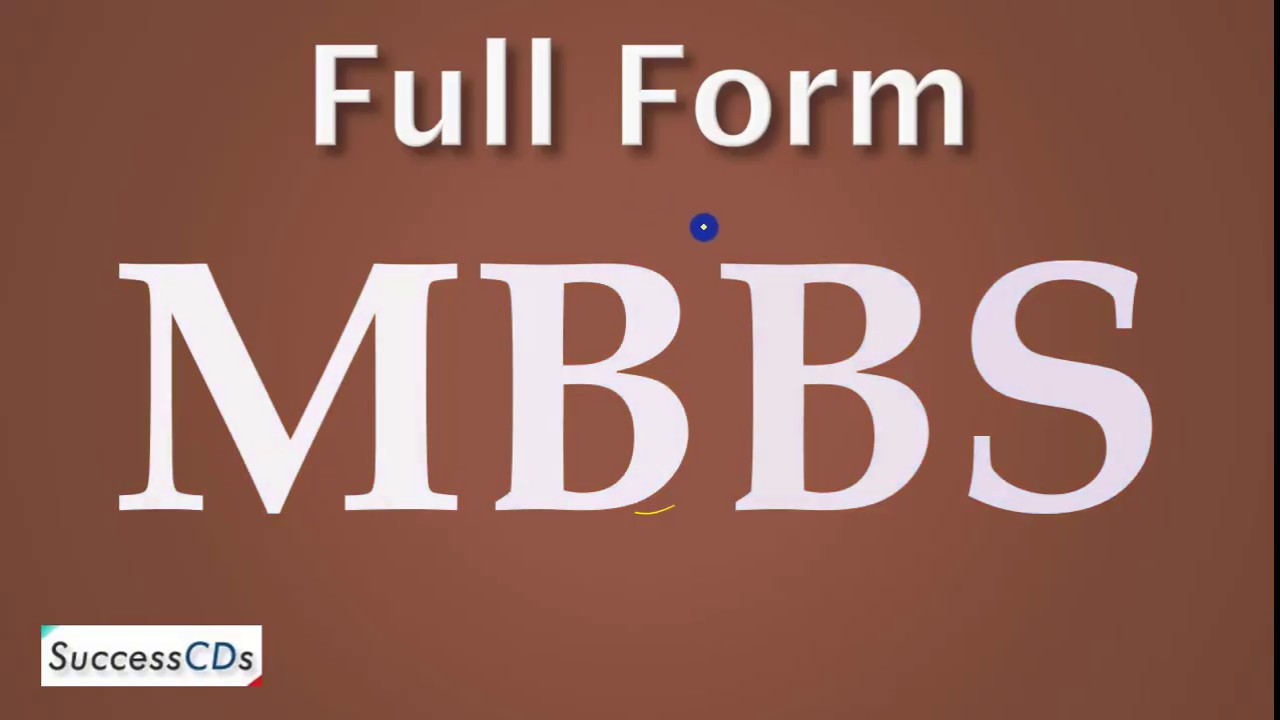
Full Form of MBBS | mbbs full form
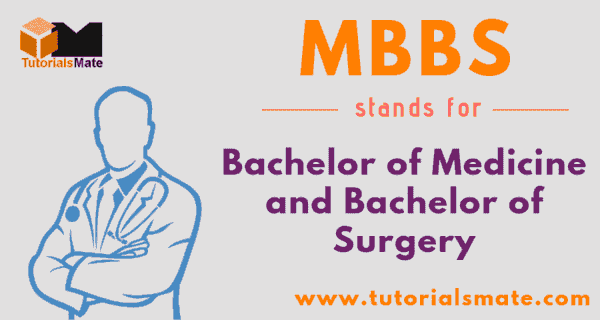
MBBS Full Form: What is the full form of MBBS? – TutorialsMate | mbbs full form